Road map of an industrial Ph.D. at Agaetis
Mid-April 2021, I started an industr ial Ph.D. (CIFRE) funded by Agaetis and at LIMOS on the subject of data quality evaluation. Industrial Ph.Ds. are funded by companies that benefit from their contribution to companies’ industrial development, and the Ph.D. candidate divides their time between the company and their lab. My time is divided equally between Agaetis and Limos, with 2-3 days a week spent on each location.
Why is data quality important ?
The aspect of data quality I’m studying is sometimes also referred to as the Garbage-In-Garbage-Out (GIGO) problem. The idea behind this name is that even a very good machine learning model won’t give useable results if the data used to train it is of bad quality. It’s therefore crucial to be able to assess data quality. However, doing so usually requires the input of an expert in said data or complex metadata that is often unavailable or costly to obtain. Moreover, traditional metrics for data quality such as accuracy and f1-score heavily rely on the existence of good quality testing data, which doesn’t always exist.
My research work so far
The first 6 months of my Ph.D. were spent on a bibliographic study of the state of the art of data quality. This work prompted a few observations: data can hold very different types of errors (we identified 12 categories), and at various degrees of presence. This diversity calls for different approaches to the process of data cleaning and repairing. Therefore, we observe a wide array of data cleaning and repairing methods that require various metadata, ranging from simple to complex to acquire.
This prompted our first research question: Is it always better to repair data? We investigated this question through 5 criteria:
- C1: the perceived difficulty of using a repairing method according to experts ;
- C2: the impact of the degradation of data on classification tasks ;
- C3: the impact of the type of error present on classification tasks ;
- C4: the effectiveness of the repairing too l ;
- C5: the impact of the classification model used.
The ins and outs of this study are presented in a more detailed version in the
paper
we presented at the conference
IDEAL 2022
(published in its proceedings). In this paper, we proposed an evaluation process that breaks down repairing methods into elementary tasks describing the actions executed to apply them (C1), including creating the metadata needed to use them. Given an error type and a repairing method, we build a tree detailing the steps of the repairing method. We then populate this tree with elements from other repairing methods for this error type, and iterate with different trees for each error type. To quantify the difficulty of each elementary task, we then asked a panel of 8 industry data scientists to rank them on a 4 values scale: easy, medium, medium+, and hard. We registered the weighted average of each elementary task as its difficulty score. We then used those weighted averages to compute difficulty scores for the whole repairing method.
To study criteria C2 to C5, we conducted an experiment where we deteriorated dataset by injecting known percentages of specific error types, to observe how these changes would affect classification task accuracies and F1 scores on various machine learning models.
To go back to our first research question: Is it always better to repair data? We found there is no answer covering all cases, but we were able to answer this question for specific cases (mainly for very low and very high error percentages). Moreover, our work on measuring the difficulty to use a repairing method provides a useful tool for decision-making when the repairing process to follow is unclear.
Opportunities working towards a Ph.D. brought me
I was also able to present my work to industry clients working in collaboration with data scientists at Agaetis. This was an interesting experience as it helped me contextualize my work into concrete applications and perspectives. I also taught an introductory course to machine learning and python. Teaching was a completely new experience for me. It was very instructive as I got to go back to the basic concepts and think about how to explain them.
Future work
I am currently working on a new conference paper to present a multidimensional quality metric. The objective behind this metric is to measure data quality for classification tasks without any metadata or a perfect testing dataset. Future work could focus on studying how to assess the repairability of data.
Ressources Agaetis
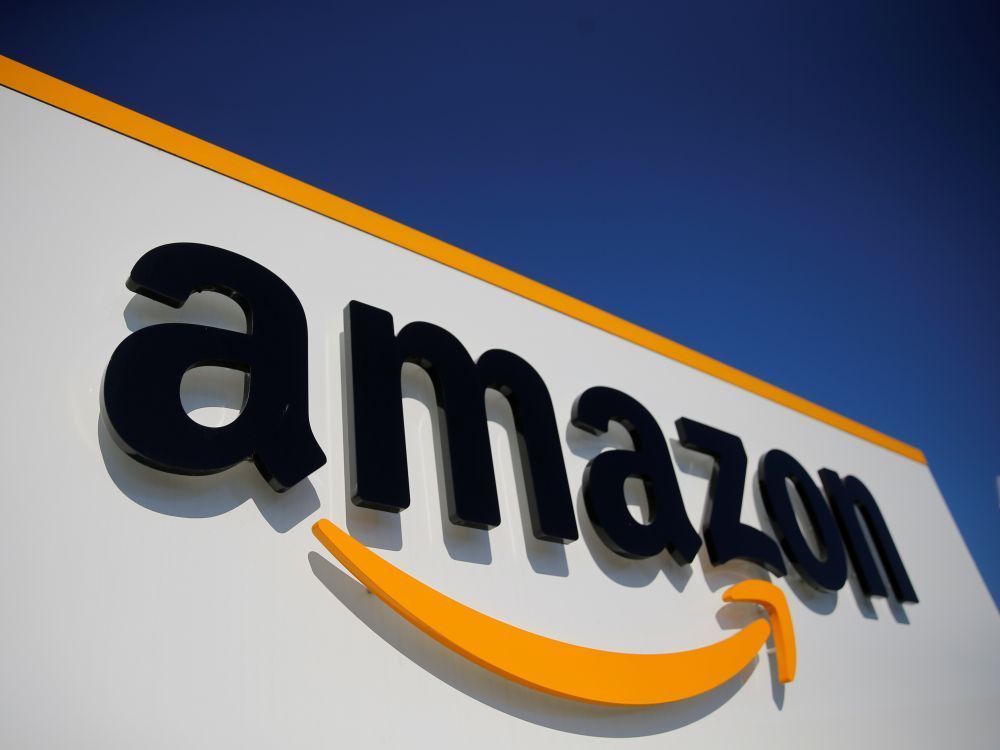
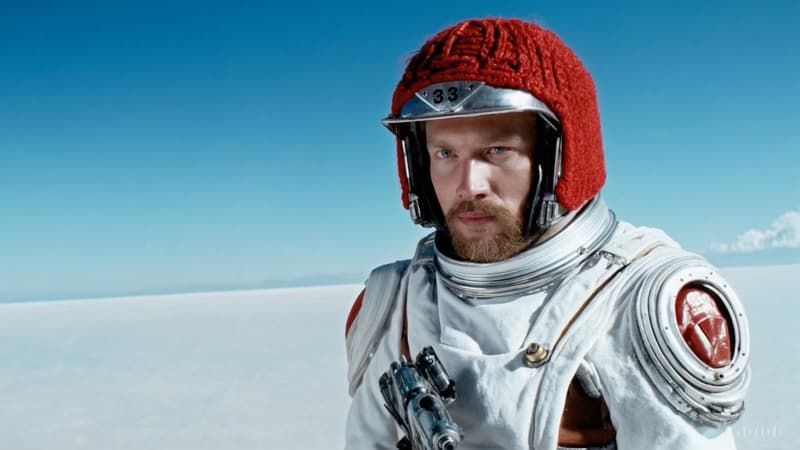